The
definition of bioinformatics is not universally agreed upon. Generally speaking,
we define it as the creation and development of advanced information and
computational technologies for problems in biology, most commonly molecular
biology (but increasingly in other areas of biology). As such, it deals with
methods for storing, retrieving and analyzing biological data, such as nucleic
acid (DNA/RNA) and protein sequences, structures, functions, pathways and
genetic interactions. Some people
construe bioinformatics more narrowly, and include only those issues dealing
with the management of genome project sequencing data. Others construe
bioinformatics more broadly and include all areas of computational biology,
including population modeling and numerical simulations. Biomedical
informatics is a slightly broader umbrella that includes not only
bioinformatics, but other areas of informatics in biology, medicine and
health-care. They are closely
related. Russ Altman "Guide to
informatics at Stanford University, 2006
Systems biology and cellular and physiological biology informatics are in this
Bioinformatics glossary
Related glossaries:
Informatics: Algorithms & data
analysis, Chemoinformatics,
Clinical
informatics Drug
discovery informatics Genomic informatics
IT
infrastructure Protein
informatics See Genomic Informatics for data analysis
using these technologies, as well as gene specific informatics. Technologies Microarrays Sequencing
Biology: DNA, Expression,
Proteins,
Sequences,
DNA & beyond
Related glossaries include Drug
Discovery & Development, Functional
genomics
Bio-IT World April 21-23 2020, Boston MA
The
Bio-IT space right now is rife with hype. Blockchain, AI, machine learning, data
science, deep learning, edge, IoT, and more are being touted as panaceas, sure
to at least facilitate a cure for what ails you. There are some legitimately
cool technologies maturing in our space, but there is also plenty of smoke and
mirrors designed to conceal the tech growing pains.
annotation:
The annotation process identifies sequence features on the contigs such as
variation, sequence tagged sites, FISH-mapped clone regions, transcript
alignments, known and predicted genes, and gene models. This stage provides
contig, RNA, and protein records with added feature annotation. In addition,
organism specific features, such as Gene Trap clones for mouse will also be
annotated.. NCBI Annotation Information 2008 http://www.ncbi.nlm.nih.gov/genome/guide/build.shtml
The value of a genome
is only as good as its annotation. At the Sanger Institute, we are providing
high quality manual curation in addition to automated prediction provided by
Ensembl. Finished genomic sequence is analysed on a clone by clone basis using a
combination of similarity searches against DNA and protein databases as well as
a series of ab initio gene predictions. Manual Curation of the Human
Genome, Wellcome Trust, Sanger Institute, 2003
https://www.sanger.ac.uk/science/groups/vertebrate-annotation
archived Each fragment of DNA contains unique features. A DNA fragment may encode a
portion of a gene or a gene control sequence, or the fragment may be a portion
of a
genome that has no apparent function. Bioinformaticists perform detailed
analysis of DNA fragments, comparing new DNA sequence, previously annotated DNA
sequences and identifying common characteristics, and assigning known or
putative potential functions to the DNA sequence. Cross species DNA sequence
comparison is quite common and can reveal common genes shared between organisms.
A bioinformatic study may also require peptide to peptide comparisons allowing
common structural features of proteins to define the function a DNA fragment
encoding a specific protein or enzyme. Explanatory notes, comments, analysis and commentaries added to a database.
May refer to sequence data or protein structures and includes predictions, characterizations,
summaries, and other detailed information, including gene function. Annotation can be manual (as in SWISS- PROT) or automated (as in TrEMBL).
Since annotation is highly skilled and labor intensive, efforts are being
made to automate the process, at least for preliminary data. Related terms: annotated databases, curated databases, comparative genome annotation,
distributed annotation system, genome annotation; SNPs
& genetic variations Genetic Annotation Initiative Narrower
terms: baseline annotation, computational annotation, distributed sequence
annotation; Proteomics: annotation - proteins
big
data:
Bioinformatics for Big Data
March 11-13, 2019 • San Francisco, CA
Program | Creating
Clinically Actionable Data
In the era of precision medicine, enormous amounts of data are being
generated from disparate sources including omics, imaging, sensing
and beyond. Today, computational scientists need to develop better
tools to manage, integrate and share data to make it clinically
actionable
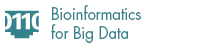
the term "Big
Data" is meant to capture the opportunities and address the
challenges facing all biomedical researchers in releasing,
accessing, managing, analyzing, and integrating datasets of diverse
data types. Such data types may include imaging, phenotypic,
molecular (including –omics), clinical, behavioral, environmental,
and many other types of biological and biomedical data. They may
also include data generated for other purposes (e.g., social media,
search histories, and cell phone data). The datasets are
increasingly larger and more complex, and exceed the abilities of
currently-used approaches to manage and analyze them. Biomedical
Big Data primarily emanate from three sources: 1) a few groups that
produce very large amounts of data, usually as part of projects
specifically funded to produce important resources for the research
community; 2) individual investigators who produce large datasets
for their own projects, which might be broadly useful to the
research community; and 3) an even greater number of investigators
who each produce small datasets whose value can be amplified by
aggregating or integrating them with other data. Centers of
Excellence for Big Data Computing in the Biomedical Sciences (U54),
July 2013 http://grants.nih.gov/grants/guide/rfa-files/RFA-HG-13-009.html
BioConductor:
An open source and open development software project to provide tools for the
analysis and comprehension of genomic data (bioinformatics). http://www.bioconductor.org/
Bioinformatics
April 17-18, 2019 • Boston, MA | case
studies using computational resources and tools that take data from multiple
–omics sources and align it with clinical action. Turning big data into smart
data can lead to real time assistance in disease prevention, prognosis,
diagnostics, and therapeutics. With the ever-increasing volume of information
generated for curing or treating diseases and cancers, bioinformatics
technologies, tools and techniques play a critical role in turning data into
actionable knowledge to meet unstated and unmet medical needs.
https://www.bio-itworldexpo.com/bioinformatics
The Bioinformatics and
Computational Biology program, which supports the National Centers for
Biomedical Computing, aims to develop novel, cutting-edge software and data
management tools to effectively mine the vast wealth of biomedical data
generated from sophisticated modern laboratory techniques and facilitate data
sharing between researchers. NIH Common Fund http://commonfund.nih.gov/bioinformatics/index.aspx
Roughly, bioinformatics describes any use of computers to handle biological information. In practice the definition used by most people is narrower; bioinformatics to them is a synonym for "computational molecular
biology" - the use of computers to characterise the molecular components of living things.
Damian Counsell, bioinformatics.org FAQ] http://bioinformatics.org/faq/#whatIsBioinformatics
See
above bioinformatics.org FAQ for tight and loose definitions of bioinformatics,
and information on how long the term has been used.
We have coined the term Bioinformatics for the study of informatic processes
in biotic systems. Our Bioinformatic approach typically involves spatial, multi-
leveled models with many interacting entities whose behavior is determined
by local information. Theoretical Biology Group, Univ. of Utrecht, Netherlands,
Paulien Hogeweg Director http://www-binf.bio.uu.nl/
Original definition was “the study of informatic processes in biotic
systems” Paulien Hogeweg MIRROR beyond MIRROR, puddles of LIFE, in Artificial
Life, ed. C.G. Langton, Addison Wesley, 297-316, 1988 http://en.wikipedia.org/wiki/Paulien_Hogeweg
The earliest Medline reference I've found to bioinformatics is William Bain's
"Bioinformatics in Europe - the federation strikes back" in
Trends in Biotechnology 11(6): 217- 218 June 1993.
Narrower terms: bacterial bioinformatics, comparative bioinformatics, functional bioinformatics,
glycobioinformatics, medical bioinformatics, molecular bioinformatics, pharmaceutical bioinformatics,
protein bioinformatics; Protein informatics structural bioinformatics; Related terms: European
Bioinformatics Institute EBI, Open Bioinformatics Foundation; Algorithms data mining
Carole Goble, Seven
Deadly Sins of Bioinformatics, 2007 http://www.slideshare.net/dullhunk/the-seven-deadly-sins-of-bioinformatics
Bioinformatics information resources
poster Mary Chitty presented at BioIT World
2014
biojava.org:
BioJava is open-source and entirely hosted on GitHub.
You can check the code, submit issues and pull requests and download
latest and past release binaries from GitHub.
.https://biojava.org/
biological
computing:
Wikipedia
https://en.wikipedia.org/wiki/Biological_computing
biological
databases:
Biological databases have inherent complications stemming from
the nature of the information they contain and the dependence of computational
methods on these data. Most biological data are not digital, making machine-
readability of the data (for automated data- mining) impossible. In addition,
the lack of standardized nomenclature and ontology, the use of protein aliases
(leading to ambiguity), the lack of interoperability across databases, and the
presence of errors in database annotations have hindered and complicated the use
of computational methods. Defining the Mandate of Proteomics in the Post-
Genomics Era, Board on International Scientific Organizations, National Academy
of Sciences, 2002 http://www.nap.edu/books/NI000479/html/R1.html
bioMOBY:
An international group of biological data hosts, biological
data service providers, and coders whose aim is to set standards for biological
data representation, distribution, and discovery. http://biomoby.org/
BIONLP.org:
Natural language processing of biology text. Bob
Futrelle, Computer Science, Northeastern Univ., US http://www.ccs.neu.edu/home/futrelle/bionlp/
BioPax:
Biological Pathways Exchange. A collaborative effort to
create a data exchange format for biological pathway data. http://www.biopax.org/
Related terms: metabolic
pathways
bioperl.org:
An international association of developers of open
source Perl tools for bioinformatics, genomics and life science research.
We work closely with our friends and colleagues at biojava.org, biopython.org
and
bioxml.org. The Bioperl server provides an online resource for modules,
scripts, and web links for developers of Perl- based software for
life science research. http://bio.perl.org/
biopython.org:
An international association of developers of
freely available Python tools for computational molecular biology. biopython.org
provides an online resource for modules, scripts, and web links for developers
of Python- based software for life science research.
http://www.biopython.org/
biosemiotics:
according to the basic types of semiosis under
study, biosemiotics can be divided into vegetative semiotics
(also endosemiotics, or phytosemiotics),
the study of semiosis at the cellular and molecular level (including the
translation processes related to genome and the organic form or phenotype);[3][4] vegetative
semiosis occurs in all organisms at their cellular and tissue level; vegetative
semiotics includes prokaryote semiotics, sign-mediated interactions in bacteria
communities such as quorum
sensing and quorum quenching.
zoosemiotics or animal semiotics,[5] or
the study of animal forms of knowing;[6] animal
semiosis occurs in the organisms with
neuromuscular system,
also includes anthroposemiotics,
the study of semiotic behavior in humans. According to the dominant aspect of
semiosis under study, the following labels have been used: biopragmatics,
biosemantics, and biosyntactics.
Wikipedia accessed 2018 Oct 18
http://en.wikipedia.org/wiki/Biosemiotics
BISTI Consortium:
The
Biomedical Information Science and Technology Initiative is a consortium of
representatives from each of the NIH institutes and centers. ...
The mission of BISTI is to make optimal use of computer science and technology
to address problems in biology and medicine by fostering new basic
understandings, collaborations, and transdisciplinary initiatives between the
computational and biomedical sciences. http://www.bisti.nih.gov/
comparative
systems biology:
My research projects in comparative
systems biology have four main thrusts: whole-genome functional
annotation, multi-clustering of molecular profiles, cross-condition analysis
of functional genomics data, and computationally-driven design of biological
experiments. The research I am conducting with my life science colleagues in
comparative systems biology has the goal of providing precise functional
annotations to hypothetical genes in model organisms and in newly-sequenced
genomes; delineating similarities and differences in cellular networks
activated in different diseases; identifying core cellular pathways common to
response networks for multiple stresses in various model organisms; and
refining our understanding of the molecular basis of disease resistance in
plant-pathogen interactions. Research interests, TM Murali, Computer Sciences,
Virginia Tech, http://people.cs.vt.edu/~murali/research.html
Broader
term: systems biology
computational annotation:
The workshop began with a series of presentations on computational annotation
and experimental approaches to biological confirmation of functional elements in
the genomes of both model organisms and the human. Subsequent to those
discussions, NHGRI outlined its proposal for a pilot project to exhaustively
determine all functional elements in a small fraction (~1 percent) of the human
genome, Initial Inventory of Functional Elements to Identify: The participants
recommended that both protein- coding genes and non- protein- coding genes need
to be identified. For each of these, the complete (full- length) coding sequence
and all variants, as well as the transcriptional regulatory elements (e.g.,
promoters and enhancers) and post- transcriptional regulatory elements (e.g.
cis- acting RNA elements) should be described. All pseudogenes should be
identified. A number of global sequence features, such as sites of methylation,
sequence variation, evolutionary history of sequence blocks and repetitive
elements were suggested for inclusion, as were a number of chromosomal elements,
such as origins of replication, nuclease hypersensitive sites, matrix attachment
sites and histone modifications. Workshop on the Comprehensive Extraction of
Biological Information from Genomic Sequence, Bethesda, Md. July 23-24, 2002, http://www.genome.gov/10005568
computational annotation technologies:
Several
‘wet bench’ technologies and resources were discussed. These included DNA
array studies, RT-PCR/ cDNAs, in situ hybridization, chromatin
immunoprecipitation, RNAi, knockout mice, and antibody analysis of protein
function. A broad range of computational approaches were also considered to be
critical for inclusion. These included both comparative sequence analysis of
multiple genomic sequences to identify conserved elements and automated
prediction of functional elements, including coding sequences, promoters,
alternative splice variants and other highly conserved regions. The importance
of ensuring close collaboration between experimental and computational
approaches was stressed. Workshop on the Comprehensive Extraction of Biological
Information from Genomic Sequence, Bethesda, Md. July 23-24, 2002, http://www.genome.gov/10005568
computational biology: The development and application of data -
analytical and theoretical methods, mathematical modelling and computational
simulation techniques to the study of biological, behavioral, and social
systems. Biomedical Information Science and Technology
Initiative BISTI Bioinformatics at the NIH, 2000 http://www.bisti.nih.gov/
I find that people use "computational biology" when discussing that subset of bioinformatics (in the broadest sense) closest to the field of classical general biology.
Computational biologists interest themselves more with evolutionary, population and theoretical biology rather than cell and molecular biomedicine. It is inevitable that molecular biology is profoundly important in computational biology, but it is certainly not what computational biology is all about (see next paragraph). In these areas of computational biology it seems that computational biologist's have tended to prefer statistical models for biological phenomena over
physico- chemical ones. This is often wise...
One computational biologist (Paul J Schulte) did object to the above and makes the entirely valid point that this definition derives from a popular use of the term, rather than a correct one. Paul works on water flow in plant cells and points out that biological fluid dynamics is a field of computational biology in
itself - and this, like any application of computing to biology, can be described as computational biology...
Where we disagree, perhaps, is in his conclusion from
this - which I reproduce in full: "Computational biology is not a "field", but an "approach" involving the use of computers to study biological processes and hence it is an area as diverse as biology itself." Richard Durbin, Head of Informatics at the Wellcome Trust Sanger Institute, expressed an interesting opinion on this distinction in an interview on this distinction:
"I do not think all biological computing is bioinformatics, e.g. mathematical modelling is not bioinformatics, even when connected with
biology- related problems. In my opinion, bioinformatics has to do with management and the subsequent use of biological information, particular genetic information."
[Damian Counsell, bioinformatics.org FAQ,
2001] https://bioinformatics.org/faq/#definitionOfCompbiol
A field of biology concerned with
the development of techniques for the collection and manipulation of
biological data, and the use of such data to make biological discoveries
or predictions. This field encompasses all computational methods and theories
applicable to molecular biology and areas of computer- based techniques
for solving biological problems including manipulation of models and datasets.
MeSH, 1997 Related terms:
protein informatics
conceptual
biology:
As we see it, is not a distinct type of science, but rather
it has a different source: the information in databases... By logical, critical
analysis of existing facts and models, one can generate a hypothesis in which
predictions are formulated in testable terms, and then search for relevant
information among published reports of experiments that may have had a different
purpose altogether. MG Blagosklonny and AB Pardee, Unearthing the gems:
Conceptual Biology, Nature 416 (6879): 373, 28 March 2002
The iterative process
of analysing existing facts and models available in published literature to
generate new hypotheses. Julie C. Barnes, Conceptual
biology: a semantic issue and more, Nature 417(6889): 587-588, 6 June 2002
Related terms: Research
meta-analyses, meta- analysis
curated databases:
Often less complete than primary databases, but
they have less redundancy and the added value of scientific annotation;
therefore, a biologically significant sequence should be easier to find in such
a database and of greater value. Naturally, the degree of redundancy and
annotation in such a database depends on the experience, skills, aims, and
devotion of its curators. ... The only proper way to curate databases is the way groups like those that
developed OMIM [Online Mendelian Inheritance
in Man], SWISS- PROT and most commercial databases have done it — that
is, through making scientific judgments as data are cleaned up and merged.
Under the supervision of a curator. Other curated databases include LocusLink,
RefSeq, & SGD (Saccharomyces cerevisae Genome Database)
databases:
Collections of data in machine- readable form, which
can be manipulated by software to appear in varying arrangements and subsets.
Genetic information is stored in different ways in
different databases, which makes it hard to compare their holdings. So
while computational biologists are trying to improve the quality of the
databases, they are also working to build bridges between them. So
far, they have had only limited success … each database has its own Web
site with unique navigation tools and data storage formats that make such
searching difficult … programs can’t easily recognize data that are not
stored in a uniform way. Elizabeth Pennisi “Seeking Common language in a Tower
of Babel” Science: 449 Oct. 15 1999
distance functions or similarity scores:
The key issue in comparing expression
profiles is deciding what it means for two profiles to be
"similar." Mathematically, we need a function that takes two
expression profiles and calculates a similarity score. It is sometimes easier to
work with the opposite concept of distance, and people often speak of distance
functions instead of similarity scores. Many similarity or distance functions
are used in microarray work, and there is no consensus as to which one is best. Narrower terms: Euclidean distance, Pearson correlation
distributed annotation system: A client- server system in
which a single client integrates information from multiple servers. It allows a
single machine to gather up genome annotation information from multiple distant
web sites, collate the information, and display it to the user in a single view.
Little coordination is needed among the various information providers.
Biodas.org http://biodas.org/
dynamic modeling:
Mathematical
approaches to studying biological variation have changed little in several
decades. There is a need to develop new dynamic models to illuminate how systems
interact and evolve. Just as important, it is critical to study the nature of
biological and mathematical assumptions of models and statistics. Tools for
analyzing and interpreting data on the architecture of complex phenotypes should
be developed in the context of real biological information. Genetic
Architecture, Biological Variation and Complex Phenotypes, PA-02-110, May 29,
2002- June 5, 2005 http://grants1.nih.gov/grants/guide/pa-files/PA-02-110.html
Euclidean distance:
Commonly used distance function, which works by
treating each expression profile as defining a point in a multidimensional
space.
European Bioinformatics
Institute EBI,
Hinxton, Cambridge, UK.
An EMBL outstation. http://www.ebi.ac.uk/
functional
bioinformatics:
An emerging subfield of
bioinformatics that is concerned with ontologies and algorithms for
computing with biological function. Functional bioinformatics is the
computational counterpart of functional genomics ... is concerned
with managing and analyzing functional genomics data, such as gene
expression experiments and large- scale knock- out experiments. ..
emphasizes large- scale computational problems, such as problems involving
complete metabolic networks and genetic networks. Peter D. Karp
"An ontology for biological function based on molecular
interactions" Bioinformatics Ontology 16 (3): 269- 285, 2000
Related terms: Functional genomics,
Metabolic Engineering Ontologies & taxonomies
glycobioinformatics,
glycoinformatics: Glycosciences
LSID Life Sciences
Identifiers: Cover pages http://xml.coverpages.org/lsid.html
life sciences informatics:
Informatics are
essential at every step of genomics- based drug discovery and development. The
commercial landscape of life sciences information technology has changed
dramatically in recent years. Bioinformatics,
in particular, has gone through a dramatic boom/bust. While IT companies are
looking to the drug discovery and development arena as a new market opportunity,
pharmaceutical companies are faced with rising pressure to reduce (or at
least control) costs, and have a growing need for new informatics tools to help
manage the influx of data from genomics, and turn that data into tomorrow's
drugs. Key IT tools, such as high- performance computing, Web services, and
grids, are being used to improve the speed and efficiency of drug discovery and
development. True breakthroughs are still lacking, particularly in key areas
such as gene prediction, data mining, protein structure modeling and prediction,
and modeling of complex biological systems. However, most experts agree that IT
and bioinformatics are essential to reaching the improved productivity the
pharmaceutical industry craves.
molecular bioinformatics:
Conceptualizing biology in terms of
molecules (in the sense of physical- chemistry) and then applying
"informatics" techniques (derived from disciplines such as applied
math, CS [computer science] and statistics to understand and organize the
information associated with these molecules on a large- scale. Mark Gerstein
"What is Bioinformatics?" MB&B 474b3, 2001 http://bioinfo.mbb.yale.edu/what-is-it.html
molecular information theory: Schneider TD. A brief review
of molecular information theory. Nano communication networks.
2010;1(3):173-180. doi:10.1016/j.nancom.2010.09.002.
https://www.ncbi.nlm.nih.gov/pmc/articles/PMC3220916/
molecular systems biology:
An integrative discipline that seeks to explain the properties and behaviour of
complex biological systems in terms of their molecular components and their
interactions. Nature Publishing, Molecular Systems Biology aims &
scope http://www.nature.com/msb/authors/index.html#Aims-and-scope
Broader term: systems biology
NCBI National Center for Biotechnology Information:
Established
in 1988 as a national resource for molecular biology information,
NCBI creates public databases, conducts research in computational
biology, develops software tools for analyzing genome data, and disseminates
biomedical information - all for the better understanding of molecular
processes affecting human health and disease. Part of NIH. http://www.ncbi.nlm.nih.gov
non-redundant databases:
Researchers at the National Center for
Biotechnology Information (NCBI) coined the term "nr" database
(nonredundant database) to refer to a database in which the obviously
redundant entries have been merged. These entries are typically those that are
100%, character- by- character identical, and algorithms exist that can remove
such redundancy. Although such a database has less redundancy than a primary
database, a substantial amount of redundancy remains, and it can be removed only
by a curator using scientific judgment.
Many databases try to be “non-redundant”.
Unfortunately, biological data is too complex to fit a simple definition
of redundancy … Each “non- redundant” database has its own definition of
redundancy. George Church Lab, Harvard Medical School, US http://arep.med.harvard.edu/seqanal/db.html
Examples of non- redundant databases include UniGene
and SWISS- PROT,
while
DDBJ/ EMBL/ GenBank are redundant databases.
Open Bioinformatics Foundation
OPEN-BIO:
The purpose of the foundation is to act as an umbrella
organization for the various bio*.org projects that grew out of the original BioPerl
project. The goal of the foundation is to provide financial, administrative and
technical assistance for our various open source life science projects. http://open-bio.org/
Narrower terms: biojava.org, bioperl.org, biopython.org, bioxml.org
Related
term: biocorba.org
prediction: Narrower
terms: exon prediction, gene prediction,
ORF prediction, protein sequence prediction; Protein
informatics protein structure prediction; Related terms:
recognition
proprietary databases:
Fee- based, copyrighted databases
(in contrast to public databases such as those at DDBJ/ EMBL/ GenBank). Some databases charge subscription fees to commercial
organizations, with other arrangements available to non- profits.. Also
referred to as private databases. Compare: public databases
public databases: Freely
accessible databases such as GenBank/ EMBL/ DDBJ, ArrayExpress or BLOCKS.
There has been much debate about public vs. proprietary databases.
recognition:
Narrower terms: computational gene recognition, gene recognition, molecular recognition.
recognition site: Pharmaceutical
biology
spatio
temporal dynamics:
Local interactions in space can give rise to large
scale spatio temporal patterns (e.g. (spiral) waves, spatio- temporal
chaos (turbulence), stationary (Turing- type) patterns and transitions
between these modes). Their occurrence and properties are largely
independent of the precise interaction structure. They are indeed seen to
occur at many organizational levels of biotic systems. Space can be either
'real' space or a state space, e.g. 'phenotype space' in models of
speciation or 'shape space' in immunological models of shape- based
receptor interactions. We show that such spatio- temporal patterns have
important consequences for fundamental bioinformatic processes. Paulien
Hogeweg, Overview of Research 1993- 1998, Utrecht University, Netherlands,
1999 http://www-binf.bio.uu.nl/overview/node3.html
standards: Related terms: Bio-ontology Standards Group, CORBA,
Data
Model Standards Group, object protocol model OPM . EBI [European Bioinformatics
Institute] is also
working on standards. Microarrays
MAML, MGED,
MIAMI
systems biology: NIGMS
views "systems biology" as a conceptual framework for the analysis of
complex biological systems. Such systems derive from interactions among many
distinct components in varying contexts. These systems exhibit properties, such
as nonlinear dynamics and emergent behavior, that cannot easily be inferred from
studies of components in isolation. Systems biology relies on mathematical
methods and computational models to generate hypotheses and to design new
experiments. Iteration between theory and experiment is crucial. The quantity
and quality of data required for these approaches often challenge current
technologies, and the development of new technologies and cross-disciplinary
collaborations may be required. When applied to human health, systems biology
can be a powerful tool to test hypotheses relevant to health and disease,
particularly the results of therapeutic interventions.
National Centers for Systems Biology
http://www.nigms.nih.gov/Research/SpecificAreas/SysBio/Pages/default.aspx
The label
“systems biology” is pretty awful, except, of course, for the many
even worse labels that have been tried. More important is what SB seeks to
do: transform biology and health care into a rigorous, predictive science
offering a richer understanding of biology and a vastly improved approach
to drug development and medicine. SB would build on the molecular biology
revolution and elucidate the wiring diagrams (and their rules) buried in
the data. John Russell, BioIT World, Sept 2007 http://www.bio-itworld.com/issues/2007/sept/cover-story/
Systems
biology is frequently defined as the study of all of the elements in a
biological system and their relationship to one another in response to
perturbation. Advances in science and technology are enabling the
development of this emerging and cross-disciplinary field by allowing
researchers to explore how biological components function as a network in
cells, tissues and organisms. Recently, pharmaceutical companies have
begun to embrace systems approaches in an effort to better understand
physiology, pathogenic processes and pharmacological responses. This
review focuses on recent advances within three core areas of systems
biology: data collection, data analysis, and the integration and sharing
of data. Susie
Stevens and J. Rung, Advances in systems biology: measurement, modeling
and representation, Current Opinion in Drug Discovery and Development,
2006 Mar; 9(2): 240- 250.
Systems biology has been responsible for some of the most important
developments in the science of human health and environmental
sustainability. It is a holistic approach
to deciphering the complexity of biological systems that starts from
the understanding that the networks that form the whole of living
organisms are more than the sum of their parts. It is collaborative,
integrating many scientific disciplines – biology, computer science,
engineering, bioinformatics, physics and others – to predict how
these systems change over time and under varying conditions, and to
develop solutions to the world’s most pressing health and
environmental issues. This ability to design predictive, multiscale models
enables our scientists to discover new biomarkers for disease, stratify patients
based on unique genetic profiles, and target drugs
and other treatments. Systems biology, ultimately, creates the
potential for entirely new kinds of exploration, and drives constant innovation in
biology-based technology and computation.
Institute for Systems Biology
https://www.systemsbiology.org/about/what-is-systems-biology/
There
are basically two approaches to systems
biology. One has its roots in biology, the other in
systems theory. The former sees it as a way to integrate data from a variety of
sources. For the latter, the main idea is that the methods developed in those
fields might also have a useful application in biology, since engineering
sciences
have a tradition of borrowing from natures design principles. Only recently, the
prospect of 'designing' biological systems has become feasible. Currently this
is mostly done by 'improving' plants or animals by adding genes from other
organisms, but first simple from-scratch designs of biological functional
modules are starting to appear. Examples are designed cells as thermometers and
oscillators which are independent of the cell cycle. Even before all this became
possible, though, the possibility of using engineering methods to assist in
'reverse engineering nature' had a certain appeal. Glossary for Systems Biology,
Univ of Stuttgart http://www.sysbio.de/projects/glossary/Systems_Biology-2.shtml
The very nature of systems biology requires integrating data from a
variety of sources generated and interpreted by people skilled in different
areas -- engineering, computer science, biology, physics, mathematics, and
statistics. Key considerations in this process include the generation of
quantitative data, barriers in communication across departments, and
organizational challenges.
Glossary for systems biology
systems biology has
been around - under different names - for quite some time…
This is not the first attempt at systems-level analysis
of biological systems; there
have been several efforts in the past, the most notable of which is
cybernetics, or biological cybernetics,
proposed by NORBERT WIENER. As shown in the
historical review (see Chapter History), those earlier
attempts did provide solutions for special problems, but were bound
to fail as a 'real' systems biology because of the
lack of understanding of molecular biology at
the time and insufficient data due to deficiencies in measurement
techniques [31].
Today's advances in measurement, data acquisition and handling
technologies provide a wealth of new data which can be used to
improve existing models. That data can be divided into four
categories or key properties: system structures,
system dynamics, control methods,
and design methods [34].
Progress in these areas requires ``breakthroughs in our
understanding of computational sciences, genomics,
and measurement technologies, and integration of such discoveries
with existing knowledge'' [34].
(see Fig. 2.1)
http://www.sysbio.de/projects/glossary/Systems_Biology-2.shtml
Institutes for
System Dynamics and Control and for Systems Theory in Engineering of the
University of Stuttgart 2011 http://www.sysbio.de/projects/glossary/
Wikipedia http://en.wikipedia.org/wiki/Systems_biology
Narrower terms: comparative
systems biology, molecular
systems biology; hepatocyte
systems biology, semantic systems biology ; In
silico & molecular modeling applied systems biology, in silico biology ;
Metabolic engineering
signal transduction Pharmaceutical
biology integrative biology-
thresholding:
The researcher defines minimum and maximum values that
are considered reliable; measurements that are too low or too high are dropped
from the dataset or marked as unreliable. It also makes sense to subtract the
minimum value from all other measurements, because this reflects baseline noise.
This approach implicitly assumes that microarrays normally operate in the linear
part of the dynamic range, and that the transitions between the linear and flat
regimes occur abruptly. Broader term:
normalization
translational bioinformatics:
it is clear that many exciting and emerging health topics are
squarely within the scope of translational bioinformatics: cancer,
pharmacogenomics, medical genetics, small molecule drugs, and
diseases of protein malfunction. There is an unmistakable flavor of
personalized medicine here as well (genome association studies,
mining genetic markers, personal genomic data analysis, data mining
of electronic records): our molecular and clinical data resources
are now allowing us to consider individual variations, and not
simply population averages.
Altman
RB (2012) Introduction to Translational Bioinformatics Collection.
PLoS Comput Biol 8(12): e1002796., 2012
http://journals.plos.org/ploscompbiol/article?id=10.1371/journal.pcbi.1002796
Bioinformatics resources
EMBL-EBI Bioinformatics Services, European Molecular BIology Lab, European
Bioinformatics Institute http://www.ebi.ac.uk/services
How
to look for other unfamiliar terms
|
|